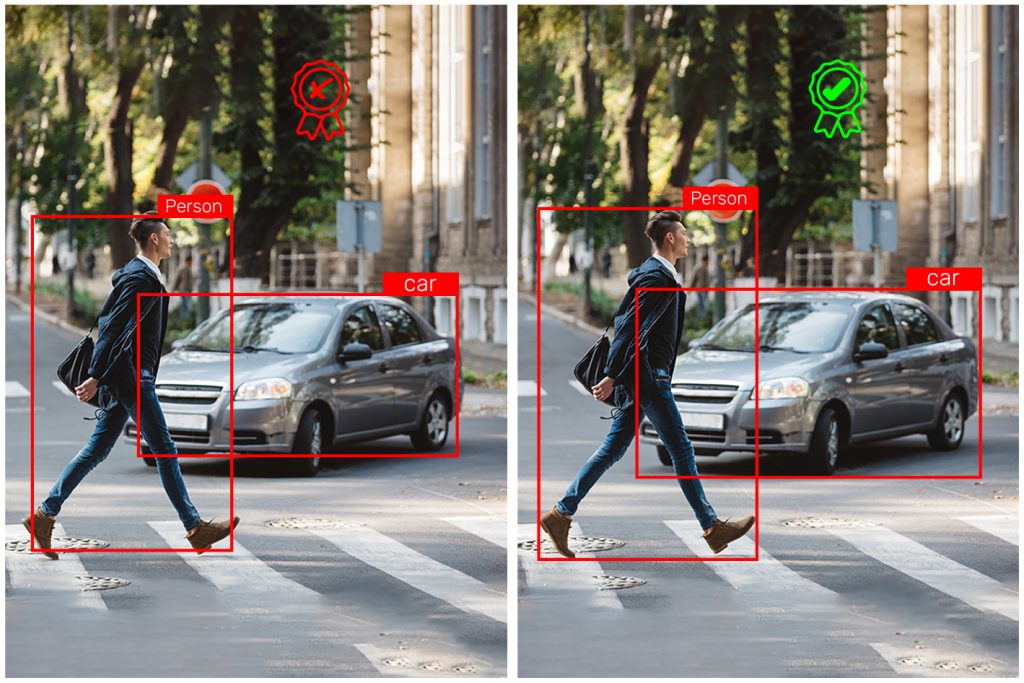
The success of an AI model is typically measured by various criteria, such as algorithms, design, implementation, etc. However, the behind the scene hero is the ‘high quality’ training data. In the current era of artificial intelligence, the demand for high quality labeled data is steadily increasing. In this blog, we will discuss this increasing demand in detail.
‘High Quality’ In Labeled Data
If a dataset meets AI training requirements and consistently yields the desired output, we can say that it is high-quality training data. However, ‘high quality’ refers to the accuracy, consistency, relevance, and reliability of the labels. AI developers possess a clear understanding of how their model should function, establishing specific guidelines for labeling the data. High-quality labeled data is defined as data that strictly adheres to these guidelines and rules.
For instance, consider an image containing various animals. If any animals are omitted from the labeling (which is required), the labeled image would be of low quality. Similarly, if any part of an animal is outside the labeled box, it is also considered poor labeling. Notably, labeling an animal that isn’t required by the client is also regarded as poor labeling. These might seem simple, but they are critical factors in defining the quality of labeled data.
Now, why should training data be error free or of high quality? Let’s discuss it in detail.
Need For High Quality Labeled Data
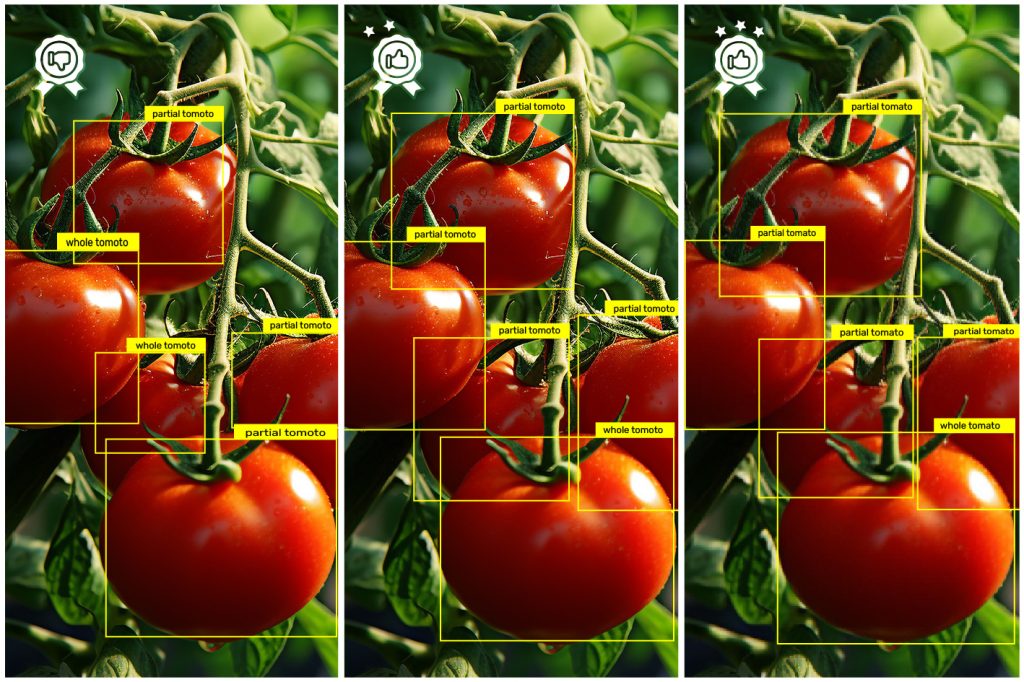
Before discussing this, we have to check what will happen if the training data is not of high quality.
The robot may remove a healthy vein during surgery.
A tool may misidentify a cancer cell as a normal cell.
An autonomous vehicle may become confused at a pedestrian crossing on the zebra crossing.
We cannot overlook these issues as mere AI performance glitches; they affect people’s lives. An accurately labeled dataset can overcome these drawbacks. Here, I will explain why this quality is so crucial.
To Get Accuracy
AI models learn by identifying patterns in the given data. If the data is full of errors, the model will learn the wrong patterns and make inaccurate predictions. For example, AI traffic cameras trained on mislabeled pictures might not be able to correctly identify ‘with helmet’ and ‘without helmet’ in a photo.
Maintain Consistency
Even if the data is generally accurate, inconsistencies can still throw off the AI model. For instance, while training an NLP model, some texts of subjects are labeled as ‘subject’ while others are labeled as ‘verb’, the model gets confused and struggles to identify subjects consistently. Inconsistent annotations confuse the model, increasing error rates and reducing reliability.
Impacting The Real World
The consequences of using poorly trained AI models can be dangerous. If a medical AI robot is trained with mislabeled data, it will be confused about how to identify tools, body parts, etc. during surgeries. In self-driving cars, poor-quality training data could lead to accidents.
Ultimately, the use of low-quality labeled data significantly hampers an AI model’s performance, affecting its accuracy, reliability, and overall usability. Models trained on high-quality labeled data are more reliable and trustworthy in real-world applications. We have to know the key reasons for increasing its demand. Let’s discuss.
Reason For Increasing The Demand
In the early days of AI, training data was often small and homogeneous. This meant that AI models could only learn to perform simple tasks on very specific data. However, today, the landscape has evolved significantly. With the widespread use of AI services, there’s an increasing demand for high-quality training data. Several key reasons contribute to this heightened demand. We can check this out in detail.
Increasing Complexity of AI Models
As AI models continue to advance, they increasingly demand more detailed and accurately labeled data to efficiently learn and perform tasks. Basic category labels are no longer sufficient for complex tasks, such as advanced natural language processing, medical image analysis, etc.
Wider Range of AI Applications
AI is being used in countless domains, such as healthcare, finance, virtual assistants, and more. Each domain requires unique and high-quality labeled data specific to its challenges and tasks. For instance, training a self-driving car demands a vast amount of high quality labeled data concerning road rules, pedestrian movements, diverse weather conditions, etc. Similarly, an NLP model requires extensive labeled text data in various languages.
Limited Access to High-quality Data
While data volume is vast, finding high-quality labeled data that meets specific needs can be challenging. This creates a high demand for reliable sources of pre-labeled data.
Increased Focus on Ethical AI
Concerns about biases, privacy, and security in AI algorithms are rising. These concerns increase the need for unbiased, labeled data to ensure ethical and responsible AI development.
The volume of training data doesn’t determine success; quality is what truly matters. Massive datasets with poor labeling fail to benefit AI models and often introduce problems. While we’re familiar with terms like ‘data labeling,’ ‘labeled data,’ etc., it’s crucial to understand the sources and methods behind obtaining high-quality labeled data.
Addressing The Demand
Primarily, three methods are commonly used for getting labeled data for AI training.
In-House Data Labeling: In-house labeling refers to conducting data labeling tasks within the organization or project team.
Crowdsourcing: Crowdsourcing involves sourcing labeled data from a large group of individuals, often through online platforms.
Outsourcing: This method involves delegating the task of labeling to external entities or specialized service providers.
Each method has its advantages and considerations concerning quality control. Regardless of the method used, data labeling primarily occurs in two ways: automated and manual data labeling. For high-quality AI training data, it is better to choose a service provider that offers manual data labeling.
Why Manual Data Labeling Matters?
Quality Control Measures
Service providers often have quality control measures in place. They implement continuous training, validation processes, cross-checking, and multiple annotator verifications to ensure the consistency and quality of labeled data.
Specialised Expertise
Service providers have specialised teams with expertise in specific domains or data types. Their knowledge and experience significantly contribute to obtaining high-quality data. Some sensitive and complex data needs human touch to get accurate labeling, which automated tools might struggle to achieve. They can also mitigate biases in data labeling, and this unbiased labeling gives high quality training data.
By outsourcing high-quality labeled data, AI researchers and developers can focus on their core work. They don’t have to worry about the training data. Concentrating on these core activities enables them to develop higher-quality AI algorithms.
Summing Up
In our daily lives, the application of knowledge governs our actions and decisions. Similarly, when a machine learns, its foundation lies in the quality of its training data—specifically, high-quality labeled data. Just as inadequate learning materials can impact our lives, any quality issues within the training data can result in system inefficiencies and errors.
Let me repeat, the quality of training data determines AI model performance. All parties involved in AI model development prioritize high-quality labeled data. The demand for this high quality will stay high. As AI expands its presence in sensitive and confidential applications, there can be no compromise regarding the quality of training data. The ongoing advancements in AI technology ensure a sustained and heightened requirement for high-quality labeled data.