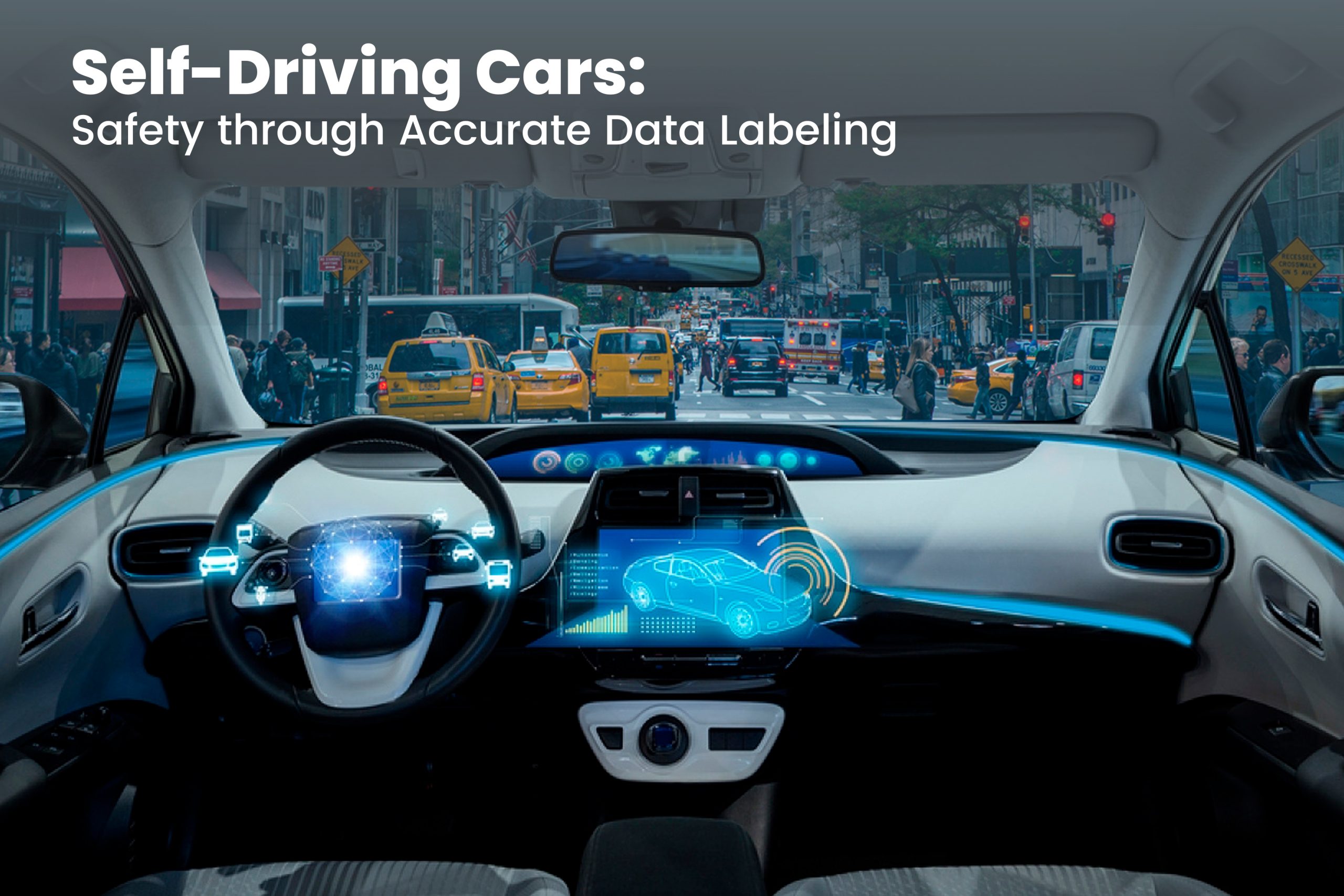
Self-driving cars are the future of transportation, promising safer roads and increased mobility for millions of people. However, recent accidents of self-driving cars have highlighted the need for more accurate data labeling to ensure the road safety. In this blog, we will explore the importance of quality and accuracy of data labeling. In the development of self-driving cars, it can help mitigate the risks associated with autonomous driving.
The Role of Data Labeling in Self-Driving Cars
Self-driving cars rely on a vast amount of data to make informed decisions while on the road. This data includes information about the environment, traffic, road conditions, and other vehicles. It also includes real-time sensory data from the car’s sensors and cameras. Machine learning algorithms use this data to learn and improve their decision-making processes. So it will enable the car to navigate safely and efficiently.
Accurate data labeling is very important to the success of self-driving cars. This involves the manual annotation of data by trained professionals. They can identify and label various objects and scenarios, such as pedestrians, traffic signs, and road markings. The labeled data is then used to train machine learning models, which can recognize these objects while driving.
The Challenges of Data Labeling in Self-Driving Cars
Data labeling for self-driving cars is a complex and challenging task that requires a high degree of accuracy and precision. This is because even small errors in data labeling can have significant consequences for the vehicle safety. For example, mislabeling a pedestrian as a stationary object could lead to a potential accident.
Another challenge is the lack of standardized data labeling guidelines, which can lead to inconsistencies in the labeling process. This can make it difficult to compare data from different sources. It can lead to errors in the training of machine learning models. To address this challenge, industry organizations such as the Autonomous Vehicle Alliance (AVA) are working on it. They are trying to develop standardized labeling guidelines for self-driving car data.
Ensuring Accuracy in Data Labeling for Self-Driving Cars
Several approaches can be used to ensure the accuracy of data labeling for self-driving cars. One is to use multiple labeling professionals to label the same data independently. This can help identify and correct errors in the labeling process, ensuring a higher degree of accuracy.
Another approach is to use advanced technology such as computer vision to automate the labeling process. This involves using machine learning algorithms to identify and label objects and scenarios automatically. It is not yet advanced enough to replace human labeling entirely to reduce errors in the labeling process.
Data Labeling for Accuracy
Data labeling is a critical component of many machine learning tasks, and it can significantly impact the accuracy of the resulting model. Therefore, to ensure accurate results, here are some important factors to take care of while data labeling:
Clear Labeling Guidelines
Clear labeling guidelines should be provided to the annotators to ensure consistency in labeling across all the data. So, these guidelines should be easy to understand and should cover all the possible scenarios that can occur during the labeling process.
Quality Control
Quality control measures should be put in place to ensure that the annotations are accurate and consistent. This can include spot-checking and monitoring, providing feedback to annotators, and revisiting previously annotated data to ensure consistency.
Labeler Training
Employees should be trained on the labeling guidelines, and they should have a good understanding of the domain and the task at hand. Training can include providing examples of annotated data and feedback on their annotations.
Quality Company Selection:
Companies for data labeling should be selected based on their expertise in the domain and their ability to follow the labeling guidelines accurately. Infolks is proud about it’s expertise in the data labeling domain.
Overall, data labeling requires careful planning and attention to detail to ensure accurate and consistent results. By taking care of these important factors, you can improve the accuracy of your machine learning model and achieve better results.
How Infolks Ensures High Quality and Accuracy in Data Labeling
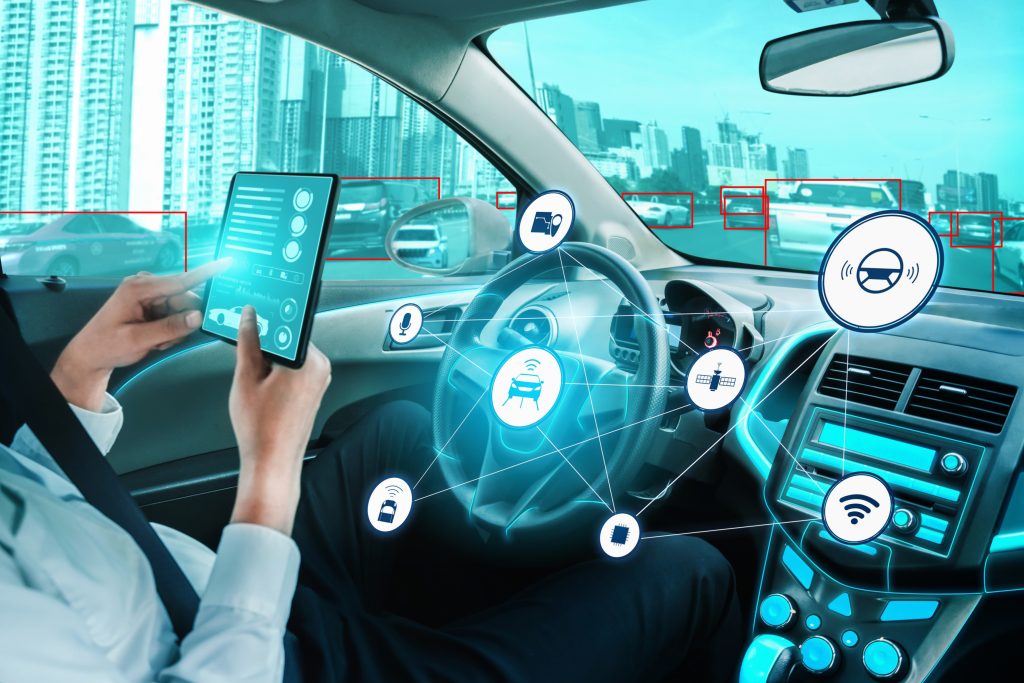
Infolks is a data labeling and annotation company that uses a triple-layer quality assurance system. Infolks and its workforce ensure the highest quality and accuracy of the output.
Data Labelers
The first level of this system is the Data Labelers. They are responsible for performing the actual task of data labeling with high accuracy and quality.
Approvers
The second level is the Approvers. They review the work done by the Data Labelers and approve it if it meets the required standards.
QC Managers
The third and final level is the QC Managers. They perform a final quality check of the labeled data. In this phase, Infolks ensure that it is accurate and of sufficient quality before delivering it to the clients.
By implementing this triple-level quality assurance system, Infolks can assure best quality in works. Infolks can keep the best output with accurate and of high quality output. So providing reliable data is important for Infolks.
Quality Day at Infolks
Infolks has designated the first day of every month as ‘Quality Day’. It is a special day for motivating and encouraging employees about the importance of maintaining quality in their work.
On this day, Infolks acknowledges and rewards its top-performing employees who have consistently demonstrated a commitment to high-quality work. Infolks aims to inspire all employees to prioritize quality in their work and deliver exceptional results to their clients.
Overall, Infolks recognizes the significance of quality in its business operations. Infolks takes proactive steps to cultivate a culture of excellence among its workforce.
Conclusion
Self-driving cars have the potential to revolutionize transportation, but their development must be approached with caution. Accurate data labeling is important to ensuring the safety of these vehicles on the road. As we continue to develop self-driving car technology, it is essential to prioritize accurate and quality data labeling. It is necessary to invest in training and guidelines to ensure consistency and accuracy in the labeling process. Only by doing so can we fully realize the potential of self-driving cars. It can transform transportation while keeping passengers and other road users safe.